Creating a transparent and resilient supply chain with analytics
Reading Time: 6 minutes
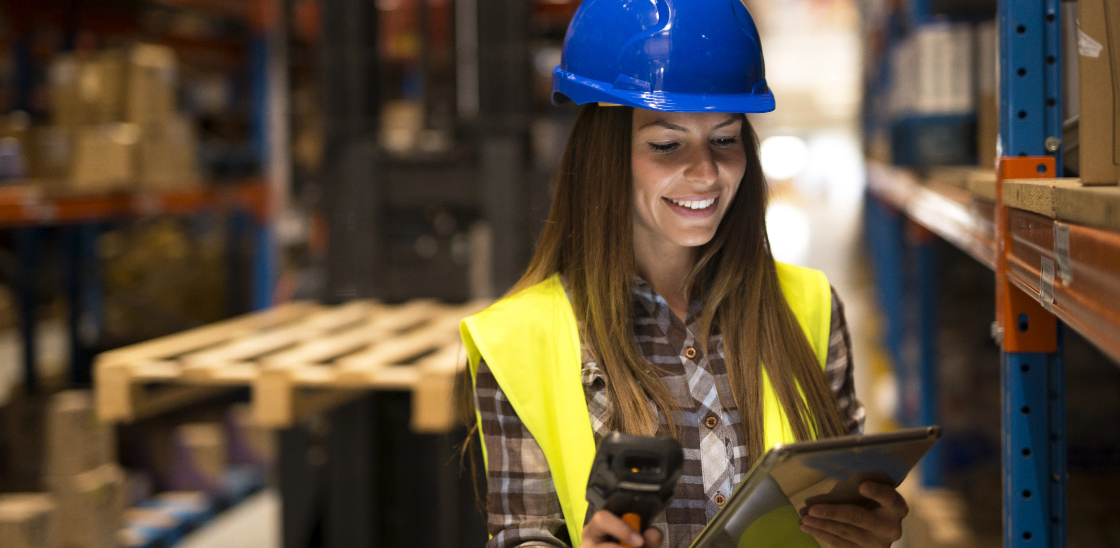
As companies start resuming operations globally following the pandemic hiatus, the impact of a prolonged period of supply chain disruption has started becoming more apparent. COVID-19 affected some of the busiest supply chains in the world and has compelled customers to embrace new lifestyles and behaviors that may well become the norm beyond the initial impact of the pandemic. A recent survey from PWC reveals that within a six-month period from October 2020 to March 2021, consumers have evolved to be more digitally mature with around 44% using a smartphone to make a purchase.
This sudden and unexpected change in customer behavior is expected to disrupt the way brands engage with customers. Companies need to look for ways to cater to customers where they are and in order to do this successfully, they will have to reinvent existing demand planning and forecasting models. The need of the hour is to increasingly monitor and minimize model forecasting errors in order to hit that sweet spot in inventory management.
The abrupt change in demand trend-based both on types of services and goods and the channels through which they are offered necessitates an increased focus from companies on channel and inventory-data visibility. Companies need to thoroughly analyze channel data from events, stores, promotions, and engagement in order to narrow down product assortments, tighten controls and gain better visibility on how to adequately stock inventory while minimizing wastage. And, it is here where supply chain analytics can help them create a difference.
Harnessing Analytics to Get More Granular Supply Chain Visibility
In reality, demand forecasting in supply chain and inventory management is already an established concept – especially in the light of the trend toward ever-growing product assortments. Traditionally, a typical inventory management strategy has revolved around holding safety stocks and creating an inventory buffer to address potential errors in forecasting or supply chain disruptions. But as the pandemic proved – this model has a downside. Sudden lockdowns meant that companies were holding excess stock which resulted in price discounts or write-downs in worst-case scenarios.
With predictive analytics-driven supply chain forecasting, companies can create a mathematical model that closely represents the demand and supply trends they are trying to understand. This may entail testing several forecasting models to select the one that most closely represents the exact situation at hand. This analytical model will simply leverage demand forecasting, historical data, and both micro and macro-economic trends to predict future events and demand trends. For this model to be successful, it’s also important for companies to harness large streams of high-quality data, since this increases the probability of an accurate forecast.
By implementing predictive supply chain analytics, companies can derive multiple benefits such as:
- Increase cash flow to accommodate the working capital reduction
- Enhance customer satisfaction by catering to diverse consumer needs
- Optimize machine utilization and worker productivity within procurement channels
- Quickly automate and optimize the entire fulfillment process
- Optimize reverse logistics to minimize costs and maintain stock
- Drive effective supply chain planning
- Reduce scraping, wastage, and damage to inventory
- Predict the exact amount of goods a facility requires to eliminate stockouts and redundant stocks
Sigmoid’s demand forecasting solution for one of its clients is an excellent example of how proper utilization of supply chain analytics can help companies drive sales, shorten demand planning cycles, reduce the risk of stock-outs and optimize inventory management.
The client – a leading cosmetics company wanted an effective demand forecasting solution that would work for diverse product categories. The existing solution did not offer a single source of information that could be consumed by different business teams. The company wanted to shorten the current forecasting cycle and make the overall process more efficient. Sigmoid enhanced their existing forecasting solution by leveraging ML algorithms to improve Weighted Absolute Percent Error (WAPE), Forecast BIAS, and coverage. The process involved removing the seasonality and trend from the products to get the base sales. The ML algorithm analyzed different data patterns such as high volatility, non-linearity, extreme values, and so on.
While the benefits of leveraging supply chain analytics are obvious, there are also several obstacles that a company may face while fully utilizing analytics. Lack of timely data, inaccurate data, and legacy data processing methods are some of the major hurdles that often undermine the success of an analytics project.
The following are some of the ways through which companies can offset the challenges mentioned above and ensure that supply chain analytics projects yield tangible results:
- To achieve tangible results from supply chain analytics, companies need to ensure that all process stakeholders get an opportunity to leverage the analytics systems. Getting buy-in from process managers and employees for analytics projects becomes seamless when the mandate comes from the top.
- In order to seamlessly implement a supply chain analytics model, companies will have to make sure that the analytics system is simple and intuitive enough for all employees to grasp. The more complex the system, the more likely it will create a barrier in usage.
- To develop the most effective supply chain analytics model, companies need to build on the requisite capabilities to collate, archive, and analyze data generated from all the key processes in the value chain.
- Time can be a defining factor in any successful analytics implementation project. Companies can get effective results only if they trust the numbers that analytics provide. One way to build trust in the analytics system is to create a closed-loop change management framework based on KPIs that accurately depict the current state of a supply chain ecosystem.
Simplifying Predictive Supply Chain Planning with Control Towers
In order to share real-time supply chain data, companies need an effective data network, bolstered by a Supply Chain Control Tower (SCCT). SCCT essentially serves as an information hub for supply chain forecasting by leveraging data intelligence and the Internet of Things (IoT). In this model, high-end sensors are used in order to track goods in real-time. Some raw materials used for manufacturing products require treatment in ideal heat. In such circumstances, smart sensors can allow companies to monitor factors like temperature, humidity, light intensity, and so on to ensure that raw materials are not exposed to harmful environmental factors. With these control towers in action, companies can easily harness analytics in real-time and gain access to actionable insights that can help.
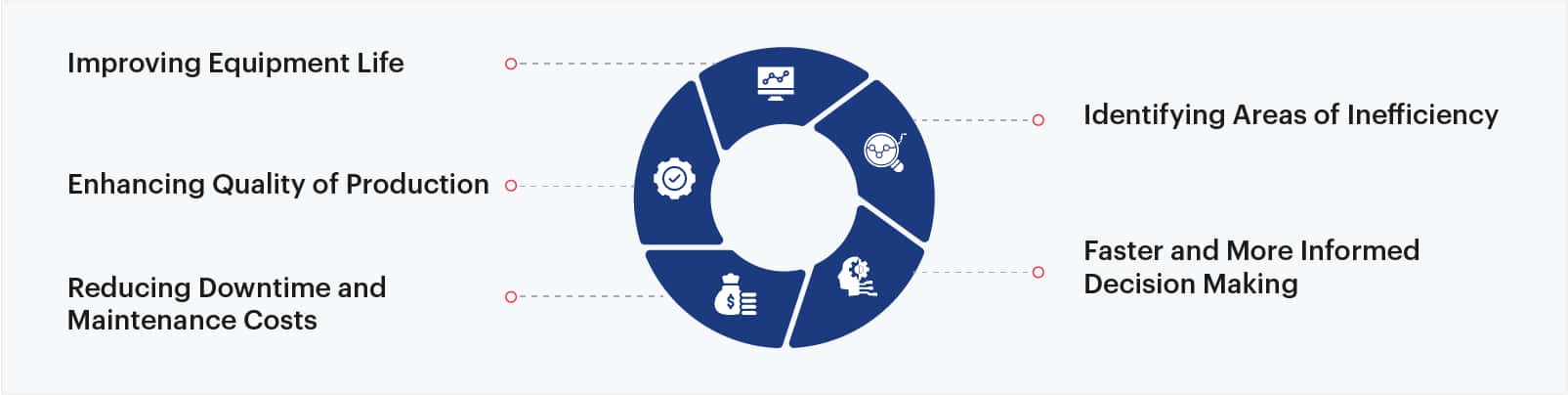
Fig: Advantages of Control Tower
IoT sensor data plays a critical role in the functioning of supply chain control towers.In this model, data interpretation errors are reduced, aggregated information is compiled faster, and predictive analysis is used to expand on that information.
Conclusion
Predictive supply chain analytics plays a critical role in helping companies minimize supply chain risks. The supply chain has several parts that interact with stakeholders. These touchpoints could be thrown out of balance by a disruption, affecting customers and ROI. To effectively manage risk, it is critical to understand when and how these risks arise. Thus, it is crucial for companies to keep everything on track with predictive analytics, which ensures complete visibility of supply chain operations.
The pandemic has come as a stress test for companies and those with a strong data foundation have adapted faster to the changes by implementing data-driven strategies that are suited for a particular situation. Even in the new normal, data and analytics will continue to fortify organizational resilience, provided companies are proactive enough to harness it.
For information on Sigmoid’s supply chain analytics solutions, please click here.
Featured blogs
Subscribe to get latest insights
Talk to our experts
Get the best ROI with Sigmoid’s services in data engineering and AI
Featured blogs
Talk to our experts
Get the best ROI with Sigmoid’s services in data engineering and AI